2020/12/18 - [2020/데이터사이언스(DL,ML)] - [CNN] CNN을 이용한 MNIST-1 // MNIST 이해 및 데이터 확인
[CNN] CNN을 이용한 MNIST-1 // MNIST 이해 및 데이터 확인
1) MNIST 데이터베이스란? MNIST 데이터베이스 (Modified National Institute of Standards and Technology database)는 손으로 쓴 숫자들로 이루어진 대형 데이터베이스이며, 다양한 화상 처리 시스템을 트레이닝..
lheon.tistory.com
1. 이미지 데이터 (X 데이터)를 3차원 데이터로 reshape
CNN의 foramt에 맞추기 위해서 3차원으로 바꿔야 합니다.
(가로, 세로, 채널) => 3차원
이 차원은 2차원이기 때문에 channel 1을 추가하여 3차원으로 바꾸어 줍니다..
(RGB 데이터라면 channel이 3개이므로 28,28,3로 표현합니다.)
X_train = X_train.reshape(60000,28,28,1)
X_test = X_test.reshape(10000,28,28,1)
2. SoftMax, One-Hot Encoding
MNIST는 output 데이터가 0부터 9까지 총 10개의 노드인
Multi-Class Classification이기때문에 마지막에 활성함수(Activation func)로 SoftMax를 사용해야 합니다.
이를 위해서 y값을 one-hot encoding 표현법으로 변환 합니다.
y데이터, 즉 라벨데이터는 원핫인코딩을 사용해야합니다.
여기서 원핫인코딩이란,
디지털 회로와 기계 학습에서 원핫(one-hot)은
단일 하이(1) 비트와 다른 모든 로우(0)를 가진 값들의 합법적인 조합이 되는 비트 그룹이다.
하나의 '0'을 제외한 모든 비트가 '1'인 유사한 구현을 일콜드라고 부르기도 한다.
통계에서 더미 변수는 범주형 데이터를 나타내는 유사한 기법을 나타낸다. - 위키백과
제가 예시를 들어보면,
만약
y값이 1일 경우 : 0, 1, 0, 0, 0, 0, 0, 0, 0, 0
y값이 6일 경우 : 0, 0, 0, 0, 0, 0, 1, 0, 0, 0
0부터 9까지 10개의 노드에서
각 값에 해당하는 노드는 1, 나머지는 0 할당하는 것입니다.
y_train = to_categorical(y_train)
y_test = to_categorical(y_test)
print(y_train[0])
[out]
[0. 0. 0. 0. 0. 1. 0. 0. 0. 0.]
위에서 확인했던 숫자5가 이렇게 5번째 index에 1로 표현된걸 확인할 수 있습니다.
3. 모델링
이제 각 Layer에대한 모델링을 해보겠습니다.
- Input 데이터의 차원, 사이즈
- [Hidden Layer ]
ㄱ) Convolutional Layer -> Conv2D(필터의 개수, 커널사이즈, 활성함수, 이름 지정 )
ㄴ) Pooling Layer -> MaxPooling2D
ㄷ) Full Conected Layer (여기서는 생략하여 차원을 축소시키는 Flatten을 사용했습니다.) - [Output Layer] -> Dense(outlayer 노드수, 활성함수 = 'softmax')
이렇게 모델링을 진행했습니다.
(딥러닝에서 히든레이어에 따라 복잡도가 달라지므로
이론적, 경험적 지식을 쌓아 최선의 방법을 찾아 가야 합니다! )
con2D의 파라미터 개수 = n_filters * (filter_size + 1)= 32*(9+1) = 320개
model = Sequential([
Input(shape = (28,28,1), name = 'input_layer'),
Conv2D(32,kernel_size=3, activation= 'relu', name = 'cov_layer1' ),
#Dropout(0.5) 데이터 기반한 weight를 줄여 오버핏팅을 줄이는 것
MaxPooling2D(pool_size= 2),
Flatten(),
Dense(10, activation= 'softmax', name = '')
])
model.summary()
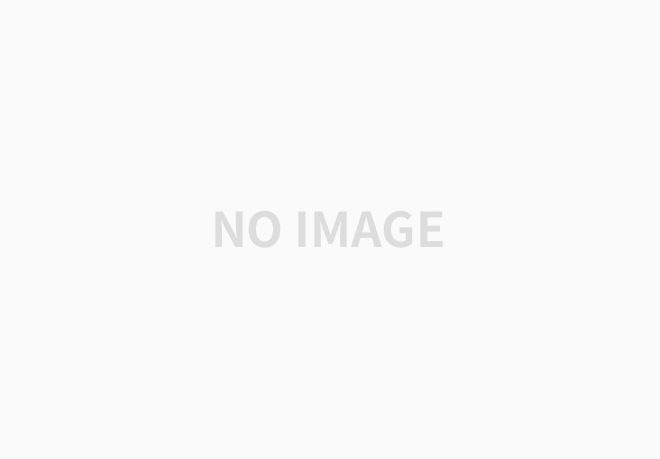
summary()함수를 통해 모델의 각 Layer와 파라미터 개수를 확인할 수 있습니다!
4. Model Compile 및 fitting
model.compile(optimizer= 'adam', loss='categorical_crossentropy',metrics=['accuracy'])
history = model.fit(X_train,y_train, validation_data=(X_test, y_test), batch_size=10, epochs = 10)
optimizer로는 CNN에서 가장 성능이 좋다고하는 adam을,
loss function은 categorical_crossentropy를 사용했습니다.
그리고 이 모든 Modeling을 가지고 fitting을 하게되면,
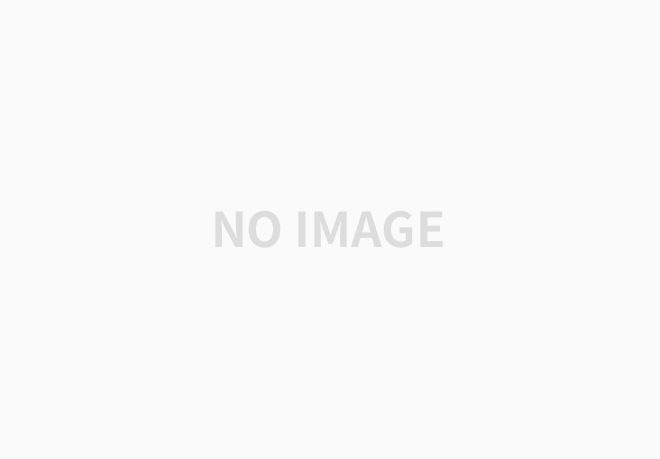
이와같이 설정한 epoch이 실행되면서
train_data) loss데이터와 accuracy
test_data) Validation loss, Validation Accuracy를 확인할 수 있습니다.
(Validation데이터는 train 데이터에서 나누어서 학습시켜야 합니다!)
그리고 이 피팅을 history에 저장하여 밑에서 그래프로 만들어 보겠습니다.
5. history 그래프 만들기 (loss, acc)
def plot_loss_curve(history):
plt.figure(figsize = (5,3))
plt.plot(history['loss'])
plt.plot(history['val_loss'])
plt.title('model loss')
plt.xlabel('epoch')
plt.ylabel('loss')
plt.legend(['train','test'], loc = 'upper right')
plt.show
def plot_accuracy_curve(history):
plt.figure(figsize = (5,3))
plt.plot(history['acc'])
plt.plot(history['val_acc'])
plt.title('model Accuracy')
plt.xlabel('epoch')
plt.ylabel('Acc')
plt.legend(['train','test'], loc = 'upper right')
plt.show
Loss 그래프, accuracy 그래프를 그리기 위해서 이처럼 함수를 설정했습니다.
그리고
plot_loss_curve(history.history)
print('train loss =', history.history['loss'][-1])
print('validation loss =', history.history['val_loss'][-1])
plot_accuracy_curve(history.history)
print('train acc =', history.history['acc'][-1])
print('validation acc =', history.history['val_acc'][-1])
print(history.history)
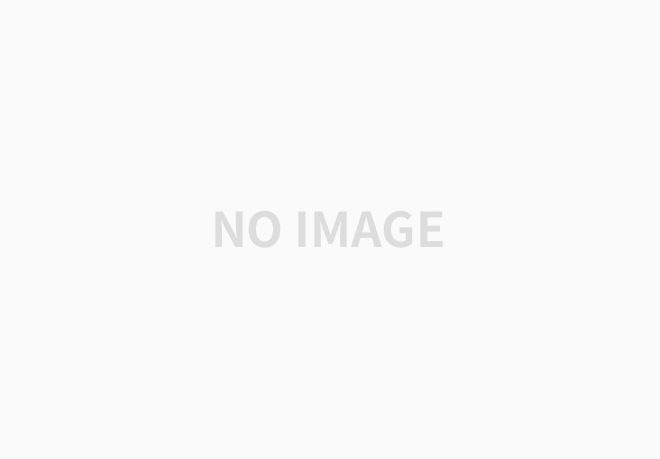
이렇게 output 결과를 얻을 수 있습니다!
조금 지저분하죠..?
이렇게 각 format으로 수정을하고 다시 출력을 해주면!
plot_loss_curve(history.history)
print('train loss ={}, validation loss = {}'.format( history.history['loss'][-1], history.history['val_loss'][-1]))
plot_accuracy_curve(history.history)
print('train Acc ={}, validation Acc = {}'.format( history.history['acc'][-1], history.history['val_acc'][-1]))
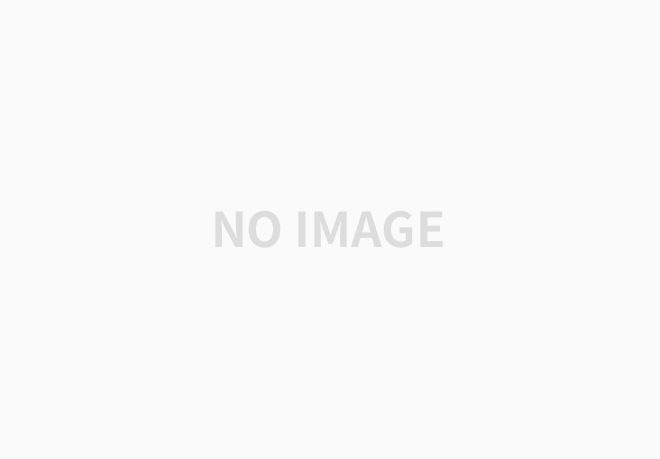
이렇게 loss 값과 accuracy값을 각 각 한줄로 확인 할 수 있습니다!
6. 모델링 정리
def train_mnist_model():
(X_train, y_train), (X_test, y_test) = mnist.load_data()
X_train = X_train.reshape(60000,28,28,1)
X_test = X_test.reshape(10000,28,28,1)
y_train = to_categorical(y_train)
y_test = to_categorical(y_test)
model = Sequential([
Input(shape = (28,28,1), name = 'input_layer_1'),
Conv2D(32,kernel_size=3, activation= 'relu', name = 'conv_layer_2' ),
MaxPooling2D(pool_size= 2,name = 'Pooling_layer_3' ),
#Dropout(0.5), 데이터 기반한 weight를 줄여 오버핏팅을 줄이는 것
Conv2D(64,kernel_size=3, activation= 'relu', name = 'conv_layer_4' ),
MaxPooling2D(pool_size= 2,name = 'Pooling_layer_5'),
Flatten(),
Dense(16, activation= 'relu', name = 'FC_layer_6'),
Dense(10, activation= 'softmax', name = 'Output_layer_7')
])
model.summary()
model.compile(optimizer= 'adam', loss='categorical_crossentropy',metrics=['accuracy'])
history = model.fit(X_train,y_train, validation_data=(X_test, y_test), batch_size=20, epochs = 3)
plot_loss_curve(history.history)
print('train loss ={}, validation loss = {}'.format( history.history['loss'][-1], history.history['val_loss'][-1]))
plot_accuracy_curve(history.history)
print('train Acc ={}, validation Acc = {}'.format( history.history['acc'][-1], history.history['val_acc'][-1]))
model.save('mnist_model')
return model
if __name__ == '__main__':
train_mnist_model()
위에서 레이어가 너무 적어 정확도가 낮게 나왔습니다.
이에 레이어에 Conv 데이터와 pooling 데이터, 그리고 Fully-connected 레이어를 추가해서
학습모델을 수정했습니다.
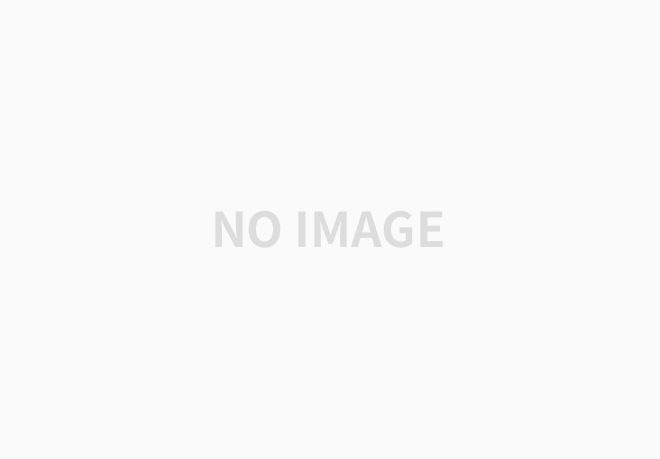
그 결과 정확도가 약 97% 나옴을 확인할 수 있습니다!
epoch가 더 증가하면 정확도가 더 증가할 것으로 생각이 됩니다 :)
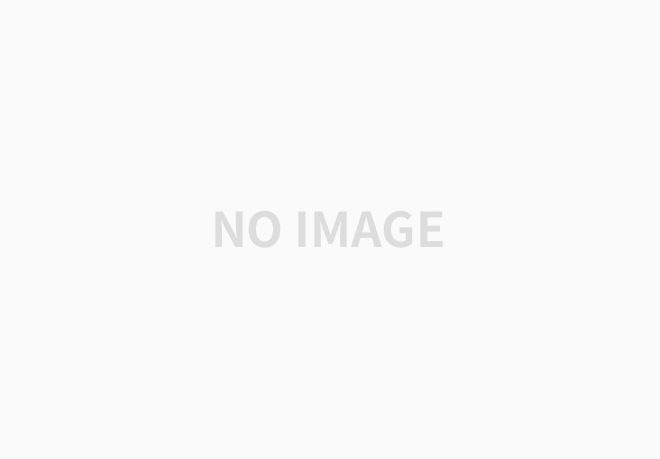
그래프에서도 1번의 epoch가 진행 된 후 급격하게 정확도가 높아지고, loss도 낮아지는걸 확인할 수 있습니다.
이렇게해서 데이터를 로딩하고, train 모델까지 완성 했습니다.
'Make the Learning Curve > Data Science' 카테고리의 다른 글
Text Mining 개요 ( Bow, VSM, TF, IDF, TF-idf , IR) (0) | 2021.04.21 |
---|---|
[CNN] CNN을 이용한 MNIST-3 // MNIST Predict (0) | 2020.12.18 |
[CNN] CNN을 이용한 MNIST-1 // MNIST 이해 및 데이터 확인 (0) | 2020.12.18 |
[딥러닝] Convolution and Pooling in CNN (0) | 2020.12.12 |
[딥러닝] Convolutional Neural Network(합성곱 신경망-CNN) 개요 (0) | 2020.12.04 |
댓글